Google AI outperforms radiologists at lung cancer detection
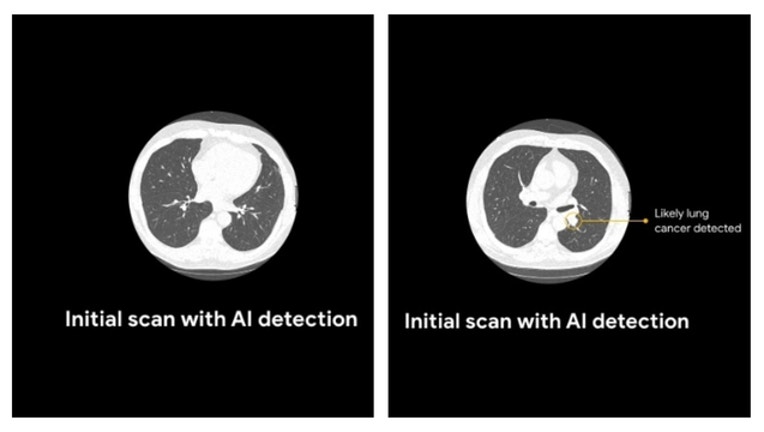
Google’s AI outperformed six trained radiologists at lung cancer detection, according to a research paper published in Nature Medicine on Monday. Photo: Google.com
San Jose, Calif. (KTVU) - Google has a couple of high-profile use-cases for AI in healthcare – in detecting eye and heart disease. In a research paper released Monday, Google has shown that its deep learning-based AI model is as good as, or better at predicting lung cancer than trained radiologists.
Google has detailed the research findings from the paper, which was published in Nature Medicine in partnership with Northwestern University. In a blog published Monday, Google said that its model performed on par or better than the six radiologists it compared the results with.
While analyzing a single computed tomography (CT) scan, Google’s AI model detected five percent more cancer cases while reducing false-positive exams by more than 11 percent compared to unassisted radiologists in the study. Where prior CT imaging was available, the model performance was on-par with the same radiologists.
To train its model, Google used 45,856 de-identified chest CT screening cases from NIH’s research dataset from the National Lung Screening Trial study and Northwestern University.
“Radiologists typically look through hundreds of 2D images within a single CT scan and cancer can be miniscule and hard to spot. We created a model that can not only generate the overall lung cancer malignancy prediction (viewed in 3D volume) but also identify subtle malignant tissue in the lungs (lung nodules),” says Shravya Shetty, M.S., Technical Lead at Google.
The model can also factor in information from previous scans to track the growth rate of suspicious lung nodules – which can be useful in predicting lung cancer risk, she adds.
Google says its collaborating with its Google Cloud Healthcare and Life Sciences team to serve this model through the Cloud Healthcare API and is in early conversations with partners to continue further clinical validation research and deployment.